Introduction
How To Interpret A Confidence Level: Interpreting a confidence level is a crucial aspect of understanding the reliability and precision of statistical results. In the realm of data analysis, a confidence level serves as a vital tool for researchers, policymakers, and decision-makers, offering insight into the confidence we can have in statistical findings. It quantifies the degree of certainty that a specific estimate, such as a population parameter or survey result, lies within a calculated range, known as the confidence interval.
In essence, the confidence level plays a pivotal role in measuring the credibility of the results obtained from a sample when making inferences about a larger population. Typically expressed as a percentage, such as 95% or 99%, it informs us how confident we can be that the true value falls within the confidence interval. The choice of a confidence level is influenced by the specific objectives of the analysis, the context, and the tolerance for potential errors.
Understanding how to interpret a confidence level is fundamental for researchers and decision-makers to navigate the complexities of data analysis. Whether in scientific research, market analysis, public policy, or various other fields, interpreting a confidence level ensures that the reliability and precision of statistical findings are taken into account, helping to guide responsible and informed decision-making.

What does it mean to interpret a confidence level?
When we create a confidence interval, it’s important to be able to interpret the meaning of the confidence level we used and the interval that was obtained. The confidence level refers to the long-term success rate of the method, that is, how often this type of interval will capture the parameter of interest.
Interpreting a confidence level in statistics is a fundamental aspect of understanding the reliability and precision of a study’s findings. A confidence level represents the degree of confidence one can have in the results obtained from a sample when making inferences about the larger population from which the sample was drawn. Commonly expressed as a percentage, such as 95% or 99%, it quantifies the likelihood that the true population parameter falls within a certain range, typically known as a confidence interval.
For example, if a study reports a 95% confidence level for a parameter estimate, it implies that if we were to draw multiple random samples from the same population and calculate a confidence interval for the parameter in each sample, we would expect the true parameter value to fall within that interval in approximately 95% of the cases. In other words, a higher confidence level signifies greater confidence in the precision of the estimate but comes at the cost of wider intervals, which might make the estimate less informative.
Interpreting a confidence level is crucial for decision-makers, researchers, and policymakers, as it helps assess the credibility of statistical results and informs them about the potential margin of error or uncertainty associated with the data.
What confidence level is at its best?
The 95% confidence level means you can be 95% certain; the 99% confidence level means you can be 99% certain. Most researchers work for a 95% confidence level.
The choice of the “best” confidence level in statistical analysis depends on the specific context, the research objectives, and the trade-off between precision and risk of making a Type I or Type II error. There isn’t a universally “best” confidence level; rather, it’s a matter of finding the one that suits the needs of the study.
Typically, a 95% confidence level is a commonly used standard in many scientific and social research contexts. It strikes a balance between precision and the risk of making a Type I error (rejecting a true null hypothesis) and a Type II error (failing to reject a false null hypothesis). It means that there is a 5% chance of observing a result that is outside the calculated confidence interval due to random sampling variation.
However, there are situations where a higher confidence level, like 99%, might be preferred. This is often the case when the consequences of a Type I error are severe, such as in pharmaceutical testing. Conversely, in exploratory or preliminary research, a lower confidence level, like 90%, might be acceptable to allow for wider intervals and more tolerance for error. In essence, the choice of the “best” confidence level depends on the specific goals, risks, and context of the study.
What is an example of a confidence level?
For example, if you are estimating a 95% confidence interval around the mean proportion of female babies born every year based on a random sample of babies, you might find an upper bound of 0.56 and a lower bound of 0.48. These are the upper and lower bounds of the confidence interval. The confidence level is 95%.
An example of a confidence level can be found in the context of political polling. Let’s say a polling organization conducts a survey to estimate the proportion of voters who support a particular candidate in an upcoming election. After surveying a random sample of 1,000 registered voters, they report a 95% confidence level with a margin of error of 3%. This means that the organization is 95% confident that the true proportion of voters supporting the candidate falls within a range of 3 percentage points above or below the reported result.
In this example, the reported result might be that 45% of the surveyed voters support the candidate. With a 95% confidence level and a 3% margin of error, it implies that the true proportion of supporters is likely to be between 42% and 48% for the entire population of registered voters. The 95% confidence level signifies that if the survey were repeated many times, 95% of the time, the true proportion would fall within this 42% to 48% range.
This confidence level provides valuable information to the public and decision-makers, indicating both the precision of the estimate and the margin of error to consider when interpreting the survey results. It helps users of the data understand the degree of certainty in the findings, which is especially important in fields like politics, market research, and public opinion studies.
How do you interpret confidence level results?
A confidence interval indicates where the population parameter is likely to reside. For example, a 95% confidence interval of the mean [9 11] suggests you can be 95% confident that the population mean is between 9 and 11.
Interpreting confidence level results is a critical step in understanding the reliability and precision of statistical findings. A confidence level, often expressed as a percentage (e.g., 95% or 99%), represents the degree of confidence that the true population parameter falls within a specific range, known as the confidence interval. To interpret confidence level results, one should consider the following:
First, consider the confidence interval: A confidence level is typically accompanied by a confidence interval. For instance, a 95% confidence level with a margin of error of 3% implies that there is a 95% likelihood that the true parameter value falls within 3 percentage points above or below the reported estimate. In other words, the interval represents the range of values within which the parameter is likely to exist.
Second, assess the level of confidence: The confidence level informs you about the reliability of the estimate. Higher confidence levels (e.g., 99%) provide greater certainty but come with wider confidence intervals, reducing the precision of the estimate. Conversely, lower confidence levels (e.g., 90%) offer more precision but carry a higher risk of making a Type I error. Interpreting the results involves striking a balance between the need for precision and the tolerance for error based on the specific context and objectives of the analysis.
Finally, consider the implications: When interpreting confidence level results, it’s important to understand that they do not guarantee the true parameter value but provide a range of plausible values with a certain level of confidence. Decision-makers and researchers should use this information to make informed judgments and decisions, acknowledging the uncertainty inherent in statistical estimation.
What is the lowest confidence level?
Confidence levels range from 1 (lowest confidence) to 10 (highest confidence).
The lowest commonly used confidence level in statistics is typically set at 90%. A confidence level is a fundamental concept in statistical analysis, representing the degree of confidence that the true population parameter falls within a specified range, known as the confidence interval. In the case of a 90% confidence level, it indicates that there is a 90% degree of confidence that the true parameter lies within the calculated interval. This implies a 10% chance that the true parameter value falls outside the interval due to random sampling variation.
The choice of a confidence level is a critical decision for researchers, analysts, and decision-makers, and it should align with the specific research context and objectives. Lower confidence levels, such as 80% or 85%, allow for more precision, as they result in narrower confidence intervals. However, they also bring a higher risk of making Type I errors (incorrectly rejecting a true null hypothesis). On the other hand, higher confidence levels, like 95% or 99%, provide greater confidence but lead to wider intervals, reducing precision.
Selecting the lowest confidence level should be done thoughtfully, weighing the need for precision against the tolerance for potential errors. The decision ultimately depends on the particular field, research goals, and the consequences of making errors, balancing the quest for reliability with the acceptance of a certain level of uncertainty inherent in statistical estimation.
What is the significance of a confidence level when interpreting statistical results?
The significance of a confidence level in interpreting statistical results lies in its role as a measure of the reliability and credibility of those results. A confidence level quantifies the degree of confidence that the true population parameter or the true effect size of a study lies within a specified range, known as the confidence interval. This level is typically expressed as a percentage, such as 95% or 99%, with a higher percentage indicating greater confidence.
When interpreting statistical results, a confidence level provides valuable information to researchers, policymakers, and decision-makers. It helps them understand the precision of the estimate and the potential margin of error associated with the data. For example, a 95% confidence level means that if the study were conducted many times, the true parameter would fall within the calculated interval about 95% of the time. This knowledge allows individuals to assess the robustness of the findings and make informed decisions based on the evidence, acknowledging the inherent uncertainty in statistical estimation.
The significance of a confidence level is twofold: it quantifies the level of confidence in the accuracy of the results, and it aids in the critical task of balancing precision with the potential for error when interpreting and applying statistical findings in various fields, including science, research, public policy, and business decision-making.
How does a higher confidence level affect the interpretation of a statistical study’s findings?
A higher confidence level in a statistical study, such as 99% as opposed to 95%, impacts the interpretation of the findings by increasing the level of certainty and reducing the risk of Type I errors. A higher confidence level implies a narrower confidence interval, meaning that the range within which the true population parameter is likely to fall becomes smaller. This reduction in the interval’s width leads to more precise estimates and a heightened degree of confidence in the results.
The interpretation of a study’s findings with a higher confidence level underscores the increased reliability of the data. For instance, a 99% confidence level suggests that there is only a 1% chance of observing a result outside the calculated interval due to random sampling variation. This heightened degree of certainty can be especially important in situations where making an error has significant consequences, such as in medical research or safety-critical industries.
However, it’s essential to recognize that a higher confidence level comes at the cost of wider confidence intervals, which can result in less precise estimates. Therefore, the choice of a confidence level should be based on the specific context, taking into account the trade-off between precision and the tolerance for potential errors. A higher confidence level bolsters confidence in the results but often at the expense of wider intervals and reduced precision, making it crucial to strike the right balance between confidence and precision when interpreting statistical findings.
Can you explain the relationship between a confidence interval and a confidence level in data analysis?
The relationship between a confidence interval and a confidence level in data analysis is straightforward but crucial to understand. A confidence interval is a range of values that statisticians use to estimate the true population parameter with a certain level of confidence. This range is calculated from the data and is associated with a specific confidence level, usually expressed as a percentage, such as 95% or 99%.
The confidence level indicates the degree of confidence or reliability that the true parameter falls within the calculated interval. For instance, a 95% confidence level means that if the same study were conducted multiple times, we would expect the true parameter to fall within the confidence interval in about 95% of those studies. Conversely, a 99% confidence level implies a higher level of confidence but results in a wider interval, as it allows for more tolerance of potential errors due to sampling variability.
The confidence level and the confidence interval are two inseparable components in data analysis. The confidence level provides a measure of how confident we can be in the range of values (the interval) that we use to estimate the population parameter. Adjusting the confidence level affects the width of the interval, as higher confidence levels lead to wider intervals and lower confidence levels produce narrower ones, allowing researchers to strike a balance between precision and the level of confidence they require in their estimates.
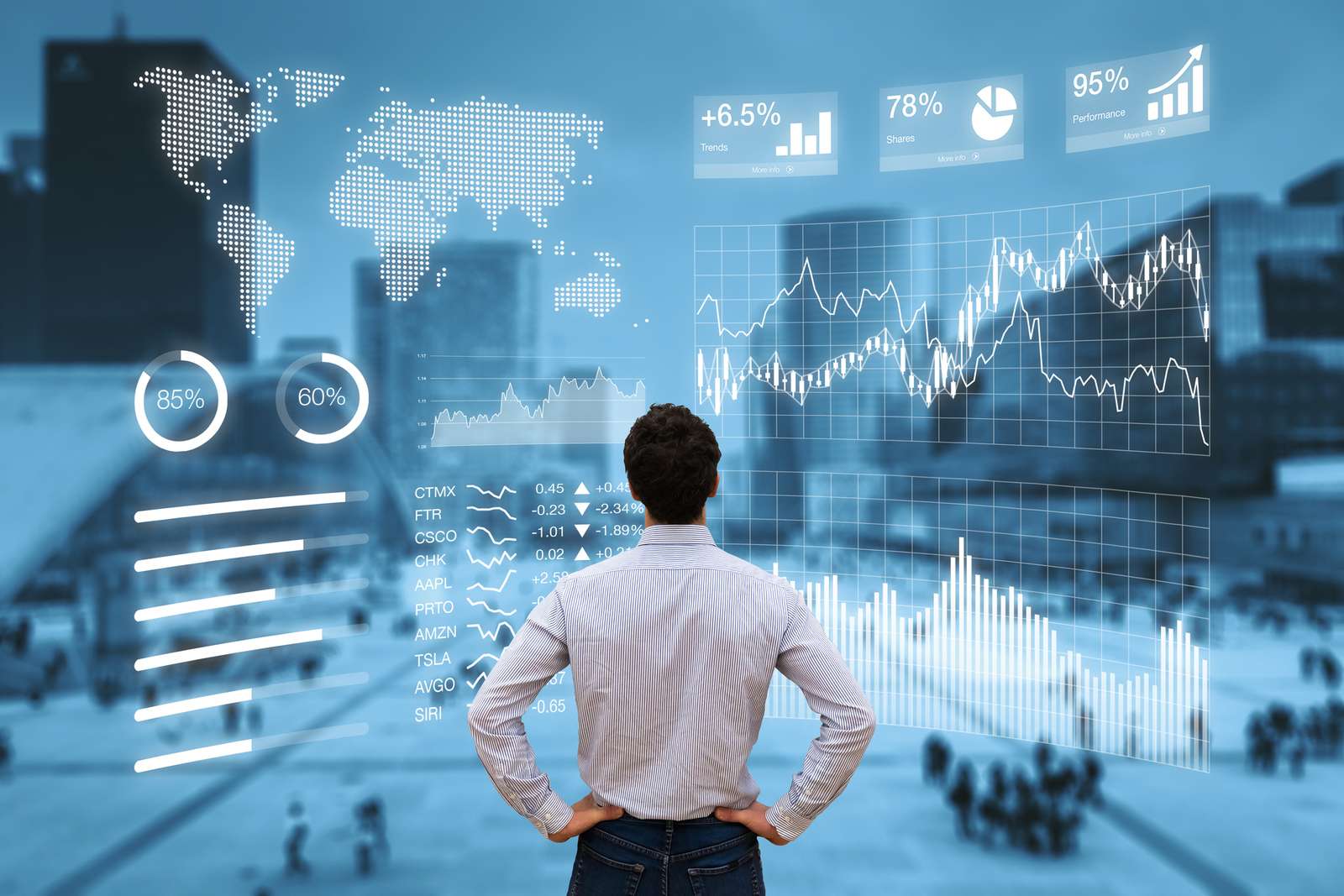
Conclusion
Interpreting a confidence level is an essential step in the process of drawing meaningful insights and making informed decisions based on statistical data. Confidence levels play a pivotal role in quantifying the reliability of results, providing a clear indication of the degree of confidence one can have in the estimated range of values for a population parameter.
The choice of a specific confidence level should be carefully considered based on the goals of the analysis, the tolerance for error, and the potential consequences of making Type I and Type II errors. While a higher confidence level offers increased certainty, it comes at the expense of wider confidence intervals and reduced precision. Conversely, a lower confidence level may provide more precise estimates but carries a higher risk of making a Type I error. The balance between these factors is essential for responsible interpretation.
In the end, a well-understood confidence level helps researchers and decision-makers navigate the complexities of data analysis, offering a valuable tool to assess the credibility of findings and make choices that are not only data-driven but also consider the inherent uncertainty in statistical estimation.