Introduction
How Do Confidence Levels Compare To Significance Levels: Confidence levels and significance levels are pivotal concepts in the realm of statistics, each serving a unique role in the interpretation and assessment of data. These two terms are often mentioned in the context of hypothesis testing and parameter estimation, and understanding their similarities, differences, and interplay is essential for researchers and decision-makers.
Confidence levels are primarily associated with parameter estimation. They represent the degree of confidence or certainty that a specific estimate, such as a population parameter or sample mean, falls within a calculated range, known as a confidence interval. These levels are expressed as percentages, such as 95% or 99%. For example, a 95% confidence level indicates that if the same study were conducted multiple times, the true parameter would be expected to fall within the confidence interval in about 95% of those instances. Confidence levels measure the precision and reliability of estimates derived from sample data, providing a sense of how well we know the true population parameter.
On the other hand, significance levels, often referred to as α (alpha), are key to hypothesis testing. They determine the threshold at which researchers consider their results statistically significant. A common significance level is 5%, signifying the maximum acceptable probability of making a Type I error (incorrectly rejecting a true null hypothesis). In hypothesis testing, the calculated p-value, which reflects the probability of observing the data if the null hypothesis is true, is compared to the significance level. If the p-value is less than or equal to the chosen significance level, it indicates statistical significance and leads to the rejection of the null hypothesis.
This article explores the relationship between confidence levels and significance levels, shedding light on how they are distinct but complementary concepts in the field of statistics. We will delve into their individual roles, how they influence each other, and the implications of their use in various data analysis contexts. By the end, you will have a clear understanding of these essential statistical tools and their impact on drawing meaningful inferences from data.
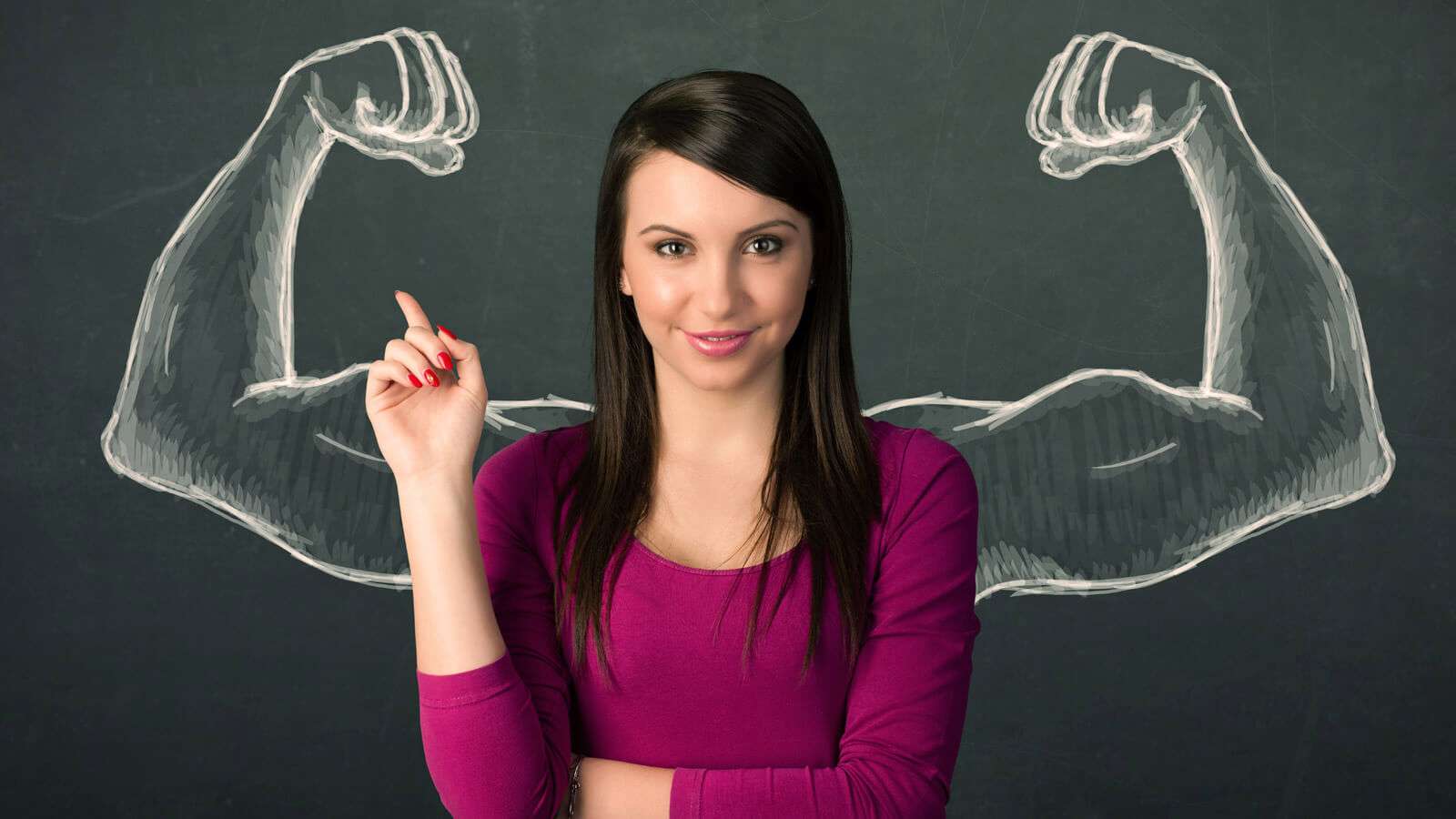
Is 95% confidence the same as 5% significance?
In accordance with the conventional acceptance of statistical significance at a P-value of 0.05 or 5%, CI are frequently calculated at a confidence level of 95%. In general, if an observed result is statistically significant at a P-value of 0.05, then the null hypothesis should not fall within the 95% CI.
No, a 95% confidence level is not the same as a 5% significance level in statistics. They represent two different aspects of hypothesis testing and statistical inference, although they are related.
A 95% confidence level indicates that you are 95% confident that the true population parameter falls within the calculated confidence interval. It quantifies the precision and reliability of an estimate or a range of values derived from a sample. In essence, if you were to take multiple samples and calculate confidence intervals, you would expect the true parameter to fall within those intervals in about 95% of the cases. This is associated with the concept of parameter estimation.
Conversely, a 5% significance level, often denoted as α (alpha), is used in hypothesis testing. It represents the maximum acceptable probability of making a Type I error (rejecting a true null hypothesis). In hypothesis testing, you compare the p-value (the probability of observing the data if the null hypothesis is true) to the significance level. If the p-value is less than the significance level, you reject the null hypothesis.
What is the level of significance for a 95% confidence of test?
For example, if your significance level is 0.05, the equivalent confidence level is 95%. Both of the following conditions represent statistically significant results: The P-value in a hypothesis test is smaller than the significance level. The confidence interval excludes the null hypothesis value.
The level of significance for a 95% confidence test, or confidence interval, is complementary to the confidence level and is typically set at 5%. In other words, a 95% confidence level and a 5% level of significance sum to 100%. These values are crucial in hypothesis testing, particularly when assessing whether a sample statistic differs significantly from a hypothesized population parameter.
In the context of a 95% confidence interval, the level of significance, often denoted as α (alpha), is the threshold used to determine statistical significance. The level of significance is the maximum acceptable probability of making a Type I error, which is the error of erroneously rejecting a true null hypothesis. Therefore, a 5% level of significance means that if the calculated p-value (the probability of observing the data if the null hypothesis is true) is less than 5%, you would reject the null hypothesis.
The relationship between a 95% confidence level and a 5% level of significance ensures that the two-sided hypothesis test is balanced. A 95% confidence interval and a 5% level of significance together allow for a reasonable degree of certainty in parameter estimation while maintaining a conservative approach to hypothesis testing. This balance helps strike a suitable compromise between estimating population parameters precisely and controlling the risk of making Type I errors.
How do confidence levels compared to significance levels?
The confidence level is equivalent to 1 – the alpha level. So, if your significance level is 0.05, the corresponding confidence level is 95%. If the P value is less than your significance (alpha) level, the hypothesis test is statistically significant.
Confidence levels and significance levels are two fundamental concepts in statistics, but they serve distinct purposes in hypothesis testing and parameter estimation.
Confidence levels are primarily used in parameter estimation and indicate the degree of confidence we have in a range of values within which the true population parameter is likely to fall. A commonly chosen confidence level is 95%, which means that if we were to repeat the process of sampling and interval estimation many times, we would expect the true parameter to be within the calculated interval about 95% of the time. Confidence levels provide a measure of the reliability and precision of estimates derived from sample data.
Significance levels, often represented as α (alpha), are central to hypothesis testing. They determine the threshold at which we consider the results of a hypothesis test to be statistically significant. A common significance level is 5%, which indicates a 5% probability of making a Type I error (rejecting a true null hypothesis). In hypothesis testing, we compare the calculated p-value to the significance level. If the p-value is less than or equal to the significance level, we reject the null hypothesis, suggesting a statistically significant result.
Confidence levels are concerned with the reliability of parameter estimates, while significance levels are used to control the risk of Type I errors in hypothesis testing. Although related, they are distinct concepts with separate roles in statistical analysis, contributing to a well-rounded and informed interpretation of data.
What do you compare the significance level to?
Compare your p-value to your significance level. If the p-value is less than your significance level, you can reject the null hypothesis and conclude that the effect is statistically significant.
The significance level in statistics is compared to the calculated p-value when performing a hypothesis test. The p-value represents the probability of obtaining the observed data or something more extreme if the null hypothesis is true. In hypothesis testing, the goal is to determine whether the p-value is less than or equal to the chosen significance level, often denoted as α (alpha).
If the p-value is less than or equal to the significance level, typically set at 5% or 0.05, it signifies that the observed data is statistically significant at the chosen level. In other words, it suggests that the data provides strong evidence against the null hypothesis, leading to its rejection in favor of the alternative hypothesis. This comparison between the significance level and the p-value forms the basis for drawing conclusions in hypothesis testing.
The choice of the significance level is crucial because it determines the threshold for declaring a result statistically significant. A lower significance level, such as 1% (0.01), makes it more challenging to declare significance, leading to a more conservative approach, whereas a higher significance level, such as 10% (0.10), makes it easier to achieve significance but may increase the risk of making Type I errors. The selection of the significance level should align with the specific research context and the acceptable trade-off between Type I and Type II errors, ensuring that conclusions drawn from hypothesis tests are both meaningful and accurate.
What is confidence level in statistics?
The confidence level is the percentage of times you expect to get close to the same estimate if you run your experiment again or resample the population in the same way. The confidence interval consists of the upper and lower bounds of the estimate you expect to find at a given level of confidence.
In statistics, a confidence level is a measure of the degree of certainty that a particular estimate or range of values includes the true population parameter. It quantifies the precision and reliability of statistical results derived from sample data. Confidence levels are typically expressed as percentages, such as 95% or 99%, and they provide information about the likelihood that the true parameter value falls within a calculated range, known as a confidence interval.
For example, a 95% confidence level implies that if the same study were conducted numerous times and confidence intervals were constructed for each sample, the true population parameter would be expected to fall within the interval in approximately 95% of those cases. In contrast, a lower confidence level, such as 90%, would provide a narrower interval but with less certainty, as it indicates a 90% confidence in capturing the true parameter.
Confidence levels are a fundamental component of statistical inference, aiding researchers and decision-makers in assessing the credibility of their results. They help strike a balance between precision and the tolerance for potential errors, allowing for responsible interpretation of data and the drawing of informed based on statistical evidence.
How do confidence levels and significance levels differ in terms of their interpretation and purpose in statistical analysis?
Confidence levels and significance levels are distinct concepts with different roles and interpretations in statistical analysis.
A confidence level is primarily related to parameter estimation. It indicates the degree of confidence we have in the range of values (confidence interval) within which the true population parameter is likely to fall. For example, a 95% confidence level means that if we were to draw many random samples and construct confidence intervals for each, we would expect the true parameter to be within those intervals about 95% of the time. Confidence levels provide a measure of the reliability and precision of estimates derived from sample data.
Significance levels, on the other hand, are central to hypothesis testing. They determine the threshold at which we consider the results of a hypothesis test to be statistically significant. A common significance level is 5%, which represents the maximum acceptable probability of making a Type I error (rejecting a true null hypothesis). In hypothesis testing, we compare the calculated p-value (the probability of observing the data if the null hypothesis is true) to the significance level. If the p-value is less than or equal to the significance level, it indicates a statistically significant result, leading to the rejection of the null hypothesis.
Confidence levels pertain to the reliability of parameter estimates, while significance levels are used to control the risk of Type I errors in hypothesis testing. They have separate roles in statistical analysis and contribute to the comprehensive interpretation of data, allowing researchers to assess both the precision of estimates and the strength of evidence against the null hypothesis.
What is the relationship between the confidence level and the significance level when testing hypotheses?
The relationship between the confidence level and the significance level in hypothesis testing is reciprocal and complementary. They represent two sides of the same statistical coin, and together they provide a comprehensive framework for making inferences based on sample data.
A confidence level and a significance level add up to 100%. For instance, if you use a 95% confidence level, it corresponds to a 5% significance level, and if you opt for a 99% confidence level, it implies a 1% significance level. This relationship ensures that hypothesis testing maintains a balanced approach.
The choice of a confidence level influences the corresponding significance level and vice versa. A higher confidence level leads to a narrower confidence interval and, consequently, a lower significance level, making it more challenging to reject the null hypothesis. Conversely, a lower confidence level results in a wider interval and a higher significance level, making it easier to declare statistical significance. This interplay between the two levels allows researchers to strike a balance between the precision of parameter estimation and the level of evidence required to reject the null hypothesis, thus tailoring the analysis to the specific goals and context of the study.
Are confidence levels and significance levels interchangeable concepts?
Confidence levels and significance levels are not interchangeable concepts in statistics, as they serve distinct and complementary purposes within the framework of hypothesis testing and parameter estimation.
A confidence level is primarily associated with parameter estimation. It represents the degree of certainty that a calculated range, known as the confidence interval, includes the true population parameter. Common confidence levels include 90%, 95%, and 99%. For example, a 95% confidence level indicates that if the same study were conducted multiple times and confidence intervals were constructed each time, we would expect the true parameter to fall within the interval in about 95% of those instances. Confidence levels are about quantifying the precision and reliability of estimates.
Conversely, significance levels, often denoted as α (alpha), are central to hypothesis testing. They determine the threshold at which researchers consider their results statistically significant. A common significance level is 5%, representing the maximum acceptable probability of making a Type I error (rejecting a true null hypothesis). In hypothesis testing, the calculated p-value (the probability of observing the data if the null hypothesis is true) is compared to the significance level. If the p-value is less than or equal to the significance level, it suggests statistical significance, leading to the rejection of the null hypothesis.
While confidence levels and significance levels are related and influence each other’s values, they have distinct roles in statistical analysis. Confidence levels focus on parameter estimation and the precision of estimates, while significance levels control the risk of Type I errors in hypothesis testing. Researchers use both concepts to ensure a comprehensive interpretation of data, balancing the need for reliable parameter estimates with the need for rigorous hypothesis testing.

Conclusion
The comparison between confidence levels and significance levels offers a comprehensive understanding of the role each plays in statistical analysis. These two concepts are integral to hypothesis testing and parameter estimation, and they work together to provide a robust framework for data interpretation.
Confidence levels are rooted in the realm of parameter estimation, offering insight into the reliability and precision of statistical estimates. They quantify the degree of confidence in a calculated range that encompasses the true population parameter. The choice of a confidence level informs researchers about the trade-off between precision and the tolerance for potential error. A higher confidence level signifies greater confidence but results in wider intervals, while a lower confidence level provides narrower intervals but with less confidence.
Significance levels, on the other hand, are the linchpin of hypothesis testing, helping researchers make decisions about the presence or absence of a statistically significant effect. They dictate the threshold for statistical significance and control the risk of making Type I errors. By comparing the p-value to the chosen significance level, researchers determine whether the results are statistically significant and whether the null hypothesis should be rejected.
These two concepts, while distinct in their applications, are intrinsically linked. The balance between them ensures that researchers can effectively draw inferences from data while considering both the reliability of parameter estimates and the rigor of hypothesis testing. This nuanced approach, considering both precision and evidence, underpins the foundation of robust statistical analysis, facilitating data-driven decision-making across various fields and industries.